A Halloween fright fest
This week I’m mostly thinking about AI ethics and algorithmic warfare. Both phrases kind of stress me out, so I guess you could consider this the Halloween edition of Metal Machine Music. Read on for some thoughts on these subjects, and a little bit of (Norwegian) history.
But first, a word of gratitude: a surprising number of people have subscribed to this newsletter since I launched it last week. If you’re one of those people, thanks for doing so. (If you’d like to become one of those people, here's the link.) I know your inbox is a sacred space (and if it’s not, I recommend making it one), so I promise to try to keep my content on point.
All power to the AI soviets
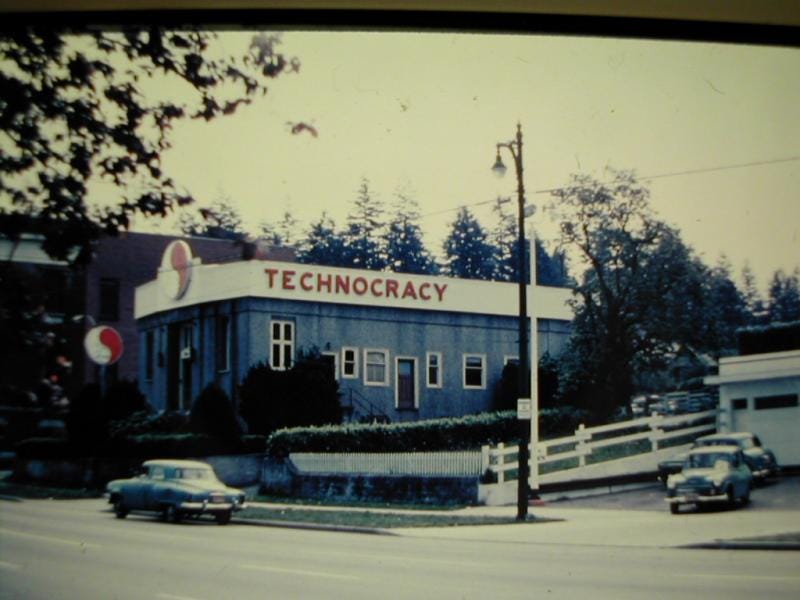
What is “AI ethics”? It’s an umbrella term for a range of initiatives currently underway in the private sector, the public sector, and the nonprofit world aimed at writing rules for the responsible development and deployment of AI systems. Some of these initiatives are cynical PR stunts. But others are well-intentioned, driven by people making good-faith efforts at averting the most dystopian outcomes.
Even so, the whole enterprise makes me deeply uncomfortable. But I wasn’t really able to articulate why until I read a paper by Daniel Greene, Anna Lauren Hoffmann, and Luke Stark entitled “Better, Nicer, Clearer, Fairer: A Critical Assessment of the Movement for Ethical Artificial Intelligence and Machine Learning.”
Greene, Hoffmann, and Stark looked at a number of high-profile “vision statements” on ethical AI and tried to figure out what they had in common. Here’s one of their conclusions (bolding mine):
Despite assuming a universal community of ethical concern, these vision statements are not mass mobilization documents. Rather, they frame ethical design as a project of expert oversight, wherein primarily technical, and secondarily legal, experts come together to articulate concerns and implement primarily technical, and secondarily legal solutions. They draw a narrow circle of who can or should adjudicate ethical concerns around AI/ML.
In other words, AI ethics is largely a top-down, technocratic affair. You substitute one layer of experts for another. Instead of the machine learning engineer, you have the AI ethicist—or, more likely, both. What’s missing from this arrangement is the people whose lives are being affected by a particular piece of software. (Or, more precisely, by a particular set of practices as structured and mediated by software.)
I’m not suggesting that nobody in the AI ethics universe is thinking about this. But as Greene, Hoffmann, and Stark make clear, actually existing AI ethics is positioned as “a project of expert oversight” in which the users and targets of technology are only intermittently consulted—and only as “stakeholders,” truly one of the worst coinages of management-speak. I’m willing to concede that a more enlightened technocracy might produce less socially harmful outcomes—it might be hard to do worse—but it’s still undemocratic.
So what would it look like to democratize AI? Pak-Hang Wong’s “Democratizing Algorithmic Fairness” offers a useful starting point, I think.
Wong argues that decisions around algorithmic fairness can’t be resolved on a technical (or technocratic) basis, because they “involve choices between competing values.” Choices about values are necessarily political. Therefore, we need political mechanisms for making these choices collectively. Wong draws on Habermas and Rawls to present a framework based on deliberative democracy: individuals come together to communicate their views, disagree, try to persuade one another, and eventually converge on a way forward that enough of them find reasonable. It’s a little too liberal for my taste—I might prefer a framework that puts more emphasis on class and conflict, less on individuals and consensus—but Wong’s basic proposition strikes me as absolutely correct. We need to politicize AI and turn it into a space of democratic decision-making.
How we might do this concretely is a bit beyond the scope of this newsletter. But here are some readings that offer materials for further thinking:
Abolition: From facial recognition software to predictive policing, there’s a lot of AI out there that simply shouldn’t exist. It deprives people of dignity and self-determination in a way that’s fundamentally incompatible with democratic values. Fortunately, there are organizers pushing for bans and moratoriums on these kinds of technologies, and winning real victories. Facial recognition bans in particular are picking up steam: three cities have prohibited local government agencies from using the technology, Bernie Sanders is calling for a ban on police use, and some state-wide measures are in the works. For a good rundown, see the AI Now Institute’s “AI in 2019: A Year in Review.” For more on the abolition approach more broadly, I’d recommend Ali Breland’s “Woke AI Won’t Save Us” from the last issue of Logic.
Into the black box: Abolition is the low-hanging fruit when it comes to democratizing AI. It takes a lot of organizing work, of course, but the demand is fairly straightforward: you’re asking for certain technologies to be eliminated. But what about when we want to keep the technology but make its functioning more democratic? That’s where things get more complicated. Here are a few promising directions:
Participatory design: This is a tradition that initially emerged from the Scandinavian labor movement in the 1970s. Unions were at the height of their power, and they started to demand more control over not just how employers were introducing new technologies into the workplace but how those technologies themselves were designed. I’m hoping to find time to research this history in greater depth—I think it would make for a good piece!—but for a quick overview, here’s a book chapter by Sara Kuhn and Terry Winograd from Bringing Design to Software (1996.)
Design justice: MIT professor Sasha Costanza-Chock has written extensively on “design justice.” Their article “Design Justice: Towards an Intersectional Feminist Framework for Design Theory and Practice” is full of interesting ideas that could be applied to the task of democratizing AI. I particularly like the “design justice principles” laid out up front, which includes this: “We believe that everyone is an expert based on their own lived experience.” To see these principles in action, see the Design Justice Zine. And look out for Costanza-Chock’s upcoming book Design Justice, which will be published in February 2020 by MIT Press.
Algorithmic Lenin: Dan McQuillan’s “People’s Councils for Ethical Machine Learning” is really worth reading. It’s one of my favorite things I’ve read on the subject. Towards the end of the piece, McQuillan proposes establishing “people’s councils” to govern the design of machine learning systems. These would be spaces of face-to-face direct democracy, but could confederate into larger structures. “It is likely that the councils operating on the same or similar topics (borders, education, or social care, for example) would confederate; that is, form regional councils based on a system of recallable deputies.” In other words: All power to the AI soviets!
Experts without technocracy: There’s a broader question here, one with ramifications far beyond AI: how can social movements (and the democratic institutions they seek to build) use experts and expertise without becoming technocratic? Yes, every cook can govern, but they might need some help from a specialist every now and then. The Black Panther Party’s healthcare activism might give us a good way to think about this: I’m hoping to read Alondra Nelson’s Body and Soul: The Black Panther Party and the Fight Against Medical Discrimination soon for inspiration.
Cloudy with a chance of drone strikes
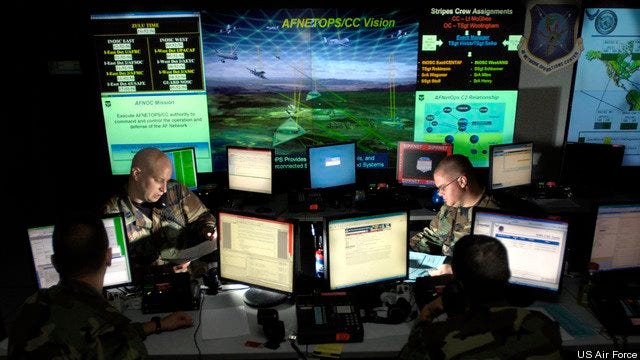
In other news: Microsoft has won the Pentagon’s $10 billion JEDI cloud contract. This is somewhat surprising, given that Amazon was widely considered the favorite. On the other hand, it’s maybe less surprising, given Trump’s hatred of Bezos. (Let them fight.)
JEDI is going to be a massive cloud computing platform that serves US forces all over the world. As I explored in a piece for the Guardian last year, the driving force behind JEDI is the Pentagon’s desire to embrace machine learning. To do so, they need to partner with the big tech firms who dominate the field. (Sorry, Raytheon.) This was starting to happen under Obama Defense Secretary Ash Carter, who set up entities like the Defense Innovation Unit and the Defense Innovation Board. But it’s accelerated in the last few years: there’s now a Joint Artificial Intelligence Center that oversees various AI projects across the Defense Department.
The idea with JEDI is simple: put all of the Pentagon’s data in a cloud (we’re talking about a lot of data), and then analyze it with cloud-based machine-learning services. There are many possible applications here, ranging from the prosaic to the lethal. But one way to think about the Pentagon’s AI push is that it’s basically an attempt to automate the forever war. AI offers a solution to the problem of how one wages war indefinitely against a vaguely (and variously) defined enemy. If I can quote myself from that Guardian piece:
The US military knows how to kill. The harder part is figuring out whom to kill. In a more traditional war, you simply kill the enemy. But who is the enemy in a conflict with no national boundaries, no fixed battlefields, and no conventional adversaries? […]
It’s one thing to look at a map of North Vietnam and pick places to bomb. It’s quite another to sift through vast quantities of information from all over the world in order to identify a good candidate for a drone strike. When the enemy is everywhere, target identification becomes far more labor-intensive. This is where AI – or, more precisely, machine learning – comes in. Machine learning can help automate one of the more tedious and time-consuming aspects of the forever war: finding people to kill.
Happy Halloween!
History corner
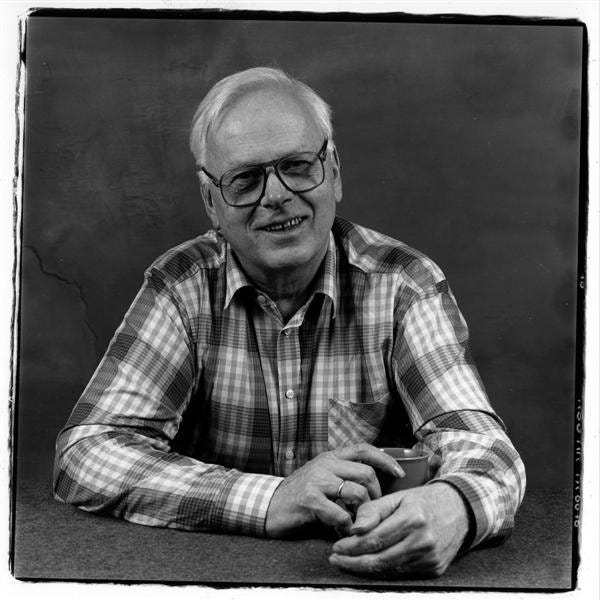
Kristen Nygaard was a Norwegian computer scientist who helped create object-oriented programming. He was also an important figure in the Norwegian labor movement and in the history of participatory design.
In the 1960s, Nygaard and another computer scientist named Ole-Johan Dahl created Simula, the first object-oriented programming language. Simula was a major breakthrough, influencing the development of important programming languages like Smalltalk, C++, and Java.
But Nygaard worried about Simula's social consequences, in particular how it might help employers discipline, displace, and deskill labor. So he began working with Norwegian unions, trying to figure out ways for workers to have greater control over technologies in their workplace. The idea was to break the employers’ monopoly on technical knowledge, and educate workers on new data systems. Towards that end, Nygaard helped make a textbook. Then, in 1974, he helped write a "data agreement"—the first of its kind in the world—that gave workers in a car tire factory the right to participate in decisions around how data systems were designed and used in their workplace.
For more on Nygaard, see the following:
Jan Rune Holmevik, “Compiling Simula”
John C. Mitchell and Krzysztof Apt, Concepts in Programming Languages
Drude Berntsen, Knut Elgsaas, and Håvard Hegna, “The Many Dimensions of Kristen Nygaard, Creator of Object-Oriented Programming and the Scandinavian School of System Development”
Knut Elgsaas and Håvard Hegna, “The Development of Computer Policies in Government, Political Parties, and Trade Unions in Norway 1961–1983”